Computational Approaches to Genetic Mechanisms in Disorders
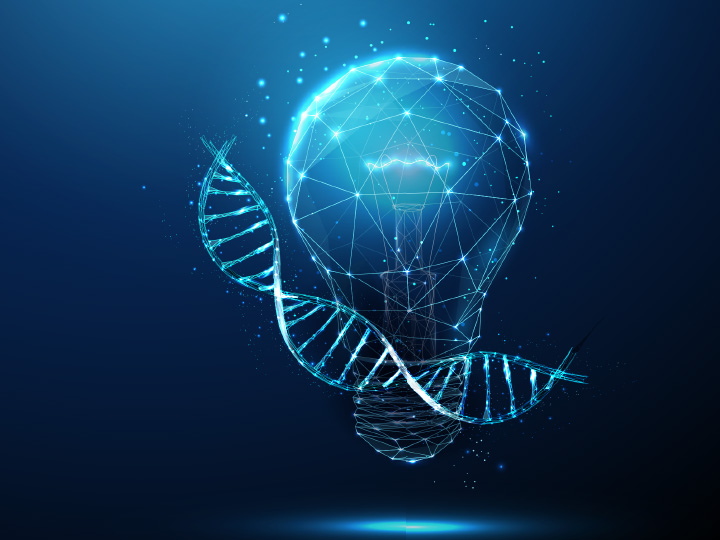
Health disparities— differences in health attributable to one’s social, racial, economic and/or environmental status— affect many nationwide. Efforts have been made in recent years to identify the root causes of these disparities. The lab of Kumaraswamy Naidu Chitrala takes this search to a molecular level, focusing on health disparities linked to aging, cancer, and neurological disorders while analyzing key genetic, proteomic, and epigenetic mechanisms using bioinformatics, computational biology, and statistical approaches.
In his talk for the HPE Data Science Institute, Chitrala first introduced his research concerning DNA methylation (DNAm), an epigenetic modification closely linked with aging and disease. Using samples collected from the Healthy Aging in Neighborhoods of Diversity Across the Life Span (HANDLS) study at the National Institutes of Health (NIH), Chitrala was able to analyze participants’ socioeconomic differences and their influence on DNA methylation and age-associated diseases, such as breast cancer, cardiovascular disease and metabolic syndrome.
In his analysis pipeline, Chitrala utilized computational tools like the Infinum MethylationEPIC BeadChip and the minfi R package to perform genome-wide DNA methylation analysis. With this, he built linear regression models to predict differences in methylation for the sample. Chitrala examined the significant results, which he used to conduct an epigenome wide association study (EWAS).
In the second half of the webinar, Chitrala touched on various other diseases and genomics-related studies within his lab, including research on PTSD, obesity, and proteomics. Some of his current lab efforts include creating a deep learning neural network to study breast cancer and metabolic syndrome genes, investigating candidate genes driving disparities among Triple Negative Breast Cancer (TNBC) patients using transcriptome association studies (TWAS), and RNA sequencing studies.